Keeping the algorithms in check
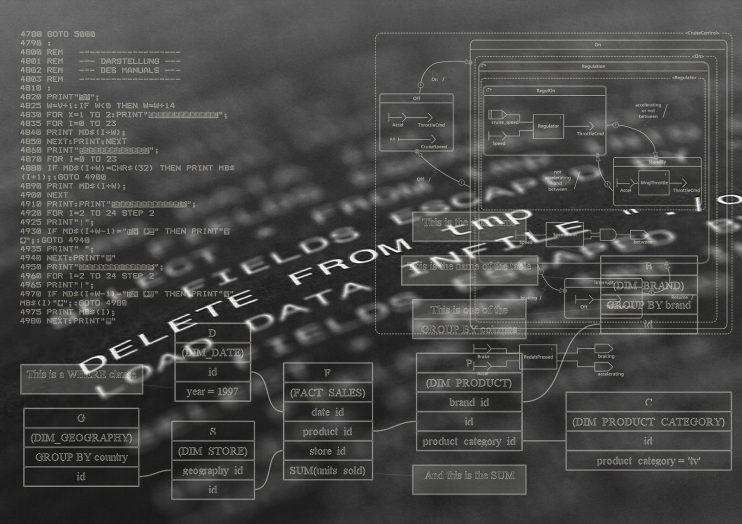
The ubiquitous adoption of algorithms is common to all sectors – in trading, lending and all consumer-facing finance there is a drive to automate.
Automation generates an enormous value opportunity and is more generally set to revolutionise finance. With minimal intervention, there will be innumerable AI algorithms making decisions that were once handled directly by human intelligence.
In this algorithmic age there is a need to safeguard society, however. There is an increasing awareness, in society and in boardrooms that algorithms can cause harm. Financial harm, such as when Knight Capital failed as a result of a glitch in its algorithmic trading system; reputational harm, such as when Amazon had to retire an AI-driven recruitment service because it showed bias against women; societal harm, such as algorithm bias in criminal sentencing, medical diagnosis, and grading of exams; among others.
From this perspective, where the last decade’s focus was on ‘data privacy’, this decade will be characterised by ‘algorithm conduct’.
The question is: what is the best way to ensure trustworthy AI? Based on our research at the UK Centre in Financial Computing (directed by Prof Philip Treleaven) and in working with tech and fintech companies, we envision a novel industry of Auditing and Assurance of Algorithms. Its task will be to validate automated and autonomous systems.
Alongside financial audit, government, business and society will soon require algorithm audit: formal assurance that algorithms are legal, ethical and safe. Because the algorithmic age is still nascent, the ‘algorithm audit’ industry will play a critical role in shaping and driving innovation, and further stimulating the burgeoning space of RegTech start-ups.
Scoring systems
Applications where auditing will be used in financial services are in every front, middle and back office of the incumbents and are the brains of the fintechs. Credit scoring systems will be audited to monitor bias and discrimination against customers with protected characteristics.
Systematic trading systems will be assessed in terms of reliability and safety when executing trades. Auditing will protect against risks from rogue algorithms. Auditing will also be used in operations. Regulators are automating their monitoring and reporting. This will lead towards fully automated compliance (in real-time and across jurisdictions).
Within computer science teams, concerns with ‘explainability’ have become central in developing AI systems. This engineering problem – explaining how and why a system works and makes decisions – bleeds into broader concerns of transparency and accountability. This is ‘AI governance’.
Systems of AI governance that are scalable and rational, which can be audited without extensive knowledge of cutting-edge engineering expertise i.e. effective AI governance, are needed.
For example, some organisations are interested in deciding whether they should buy or build a specific AI application. The framework we have developed helps them decide which option is the most suitable. Organizations can measure the key risks of a given application and determine the level of oversight needed.
By weighing these two factors, they can make the procurement process fit their needs in terms of assurance as well as having a better understanding before developing an AI application. Another case is external auditing, where an organization is interested to improve their design or reassure a third-party (internal or external) that an AI application is ‘fit-for-purpose’.
Metrics and standards
The quality of the audit will depend on the level of access the auditor will have, and in some areas and applications the metrics and standards are not settled yet.
The legislative framework for AI has not been settled. Indeed, there is a continuing and vibrant debate regarding whether new legislation, regulation and standards are required, whether existing regulation and standards can be appropriated, applied and amended, or whether self-regulation is an appropriate approach.
The juridical dimension, where national and international legislation and standards are developed concerned (particularly so for multinationals). National Financial services regulators are developing standards in collaboration with their peers.
Some things are for certain, however: lawyers must work with subject matter experts in AI or they will be left behind (or give bad advice); lawyers must engage in making projects rather than expecting to be fed legal questions by a client; lawyers need to have a grasp of how AI applications work so their suggestions are feasible and proportionate; lawyers must have a plan to be able to parse risk and explain and justify their conclusions (“explainability and the lawyer black box…” but that’s a different article); and AI and emtech work is at the sharp end of changes in legal work so those working in it gain insight into how we will all work before long …
Charles Kerrigan is a fintech partner at CMS charles.kerrigan@cms-cmno.com; Adriano Koshiyama is a Research Fellow in Computer Science at UCL and Co-founder of Holistic AI, a start-up focused on providing assurance of AI systems adriano.koshiyama.15@ucl.ac.uk; Emre Kazim is a Research Fellow in Computer Science at UCL and Co-founder of Holistic AI e.kazim@ucl.ac.uk