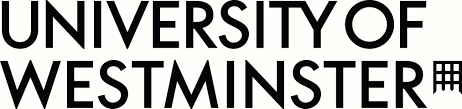
How Fintech can harness the power of big data to redefine market forecasting
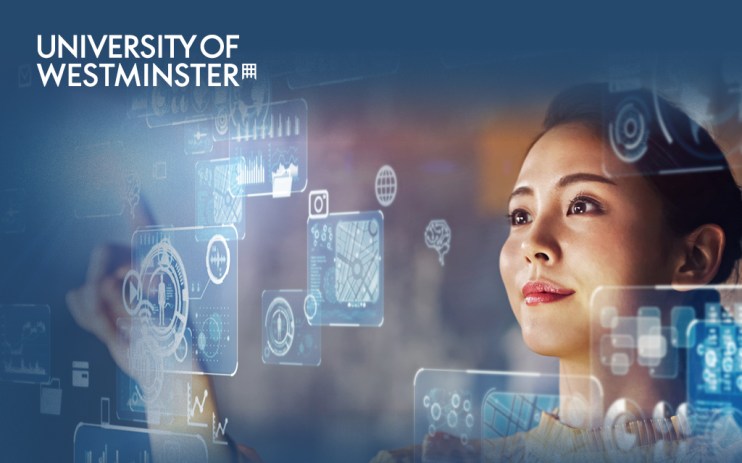
Advances in predictive analytics using machine learning (ML) algorithms and blockchain technology demonstrate how innovations in financial services are challenging traditional systems to inform decisions, improve forecasting accuracy and bring better value to customers.
As businesses seek to tap hidden intelligence within huge volumes of raw structured, semi-structured and unstructured data to support financial decision-making, ML applications are significantly contributing to markets and asset management analytics. But, in my view, feature engineering (FE) can take ML algorithms to the next level. Improving data extraction by selecting and narrowing the most important variables, FE simultaneously improves computer processing efficiencies by eliminating or reducing the importance of unimportant features.
Artificial intelligence and ML
A branch of AI, ML uses domain knowledge to organise big datasets into a series of columns called ‘features’. Mapping inputs to outputs, these features are automatically processed at high speed using computational technology and smart data analytics algorithms to deliver investment insights.
Predictive analytics embraces a wide range of statistical techniques, including ML, predictive modelling and data mining. It uses panel or sequential time series data to categorise features into discrete groups and ‘predict’ future financial outcomes.
As such, ML models provide powerful mechanisms with training datasets to generate a pattern or behaviour of past asset prices with low bias errors. But they may not be effective for out-of-sample predictability with test data: one counterproductive outcome can be to over-fit training data, which generates poor predictability scores. However, there are ways to improve the model’s forecasting abilities (lower variance errors), which we cover on our MSc Fintech with Business Analytics. A good starting point is to correctly prepare and process data using only the most important features.
Alpha generation
Value driven asset managers across the world attempt to create a bespoke portfolio of investments that regularly outperforms a benchmark index, generating alpha to inform the timing and selection of securities.
Feature engineering offers way forward
To more accurately predict future asset prices using ML techniques, feature engineering (FE) – to me, an under-rated technique – is the answer. ML algorithms written in machine code identify the most important features that drive asset prices and underweight or remove the features which are less important. FE analysis can be used on categorical and numerical data, thereby generating alpha in unseen future data.
While the pre-processing of the raw data has to be undertaken with great care, overall, FE is a refined methodology that offers the ability to understand asset valuation factors dynamically and to generate valuable alpha from unstructured and noisy raw financial datasets.
Small steps, big results
To integrate FE into a ML asset price trend analysis, the current approach involves a number of critical steps:
- The analyst approaches the initial raw data with knowledge of the key features’ specification and with a projected hypothesis, such as investment return or risk objectives (e.g., Sharpe ratio), the entire input dataset is refined. The analyst then extracts, transforms, selects and loads the input dataset for analysis.
- Following this pre-processing, input data is split into training and test groups. Using cross-validation methods, the training set is fitted to an appropriate model, e.g., a multiple regression or a polynomial regression formulae.
- Once model parameters are identified, unseen test data is fed into it and predictions are assessed for accuracy and robustness. In back-testing, the models are subjected to evaluation and further ‘fine tuning’ of feature parameters.
- If the dataset meets the accuracy score with the ML model, it is used as a predictive tool for selection of investments or asset valuations.
Importantly, this supervised learning system is not a discontinuous operation. It follows a closed loop negative feedback process as new data and information arrives in the analytical ML system. The dynamic rolling forward nature of the algorithm enables the features extraction and weightings to be continually adjusted over time, which other traditional methods of forecasting cannot do.
Fintech’s time has come
ML and FE are being deployed variously in a broad range of business problems in finance – from assessing the suitability of customers for loans to asset management. Deep Learning (DL) algorithms that utilise Artificial Neural Network (ANN) programmes in supervised, semi-supervised or unsupervised systems now mimic the human brain more closely than ever before. Blockchain technology is also evolving: in streamline banking and lending services, it mitigates counterparty risks and reliably authenticates documentation in major operational areas.
Put simply, Fintech is thriving and our MSc Fintech with Business Analytics can help you stay ahead of the game in a digitalised, transforming global economy.
Forging the future of finance
One of the top five institutions in London for Accounting and Finance (Complete University Guide 2021), the University of Westminster is a CISI Centre of Excellence in Financial Markets and CFA Institute University Affiliated Program partner. Professional accreditation of our degree and short courses includes ACCA, CBI and CIMA, covering accounting, business analytics, finance, Fintech, investment and risk finance.
Postgraduate degrees: MSc Fintech with Business Analytics, MSc Investment and Risk Finance, MSc Finance (Banking), MSc Finance (International Finance), MSc Finance and Accounting and a new MSc in Strategic and Forensic Accounting.
Ann Thapar, Principal Lecturer in Fintech, Westminster Business School