Ai: The Competitive Advantage
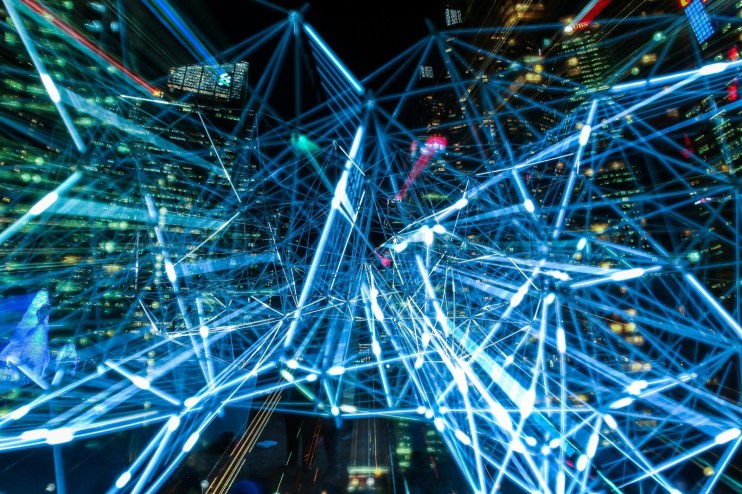
In recent years, it seems most discussions centered around technology and business innovation inevitably end up debating the future potential of Artificial Intelligence. While the longer term upside is seemingly limitless, and there is much debate about what the future of what AI might look like, what’s less understood is how AI works today – and how businesses can utilise its potential.
There is no doubt the use cases for AI are increasing year by year. This adoption is correlated with the growth in big data and the fall in the cost of deployment. Larger technology companies with proprietary data sets are leading the charge. With access to the data and the resources needed to put AI front and centre within their business models – they have a natural advantage.
Let’s consider for a moment how the tech giants are using AI in voice; Apple with Siri and Amazon with Alexa. With all the data these two companies are harvesting, their progress in these fields is exponential and gives them a long term competitive advantage over new entrants and challengers. Another example is Tesla. Once seen as a challenger car company taking on the giants with electric battery technology, another way to consider Tesla is as the market leader in automated driving. Elon Musk has talked about seeing Tesla cars as a platform that gathers driver data to train their AI. Tesla is not in the business of selling cars but AI. They are simply seeding cars to collate the data which trains their AI over time. Everytime a Tesla driver touches the wheel to make an adjustment, there is a feedback loop that is ultimately training their driverless systems. It’s about data first thinking that translates to long term competitive advantage. As a result,Tesla will one day be the proprietors of the world’s finest automated driving AI – and when they do they will be sure to charge drivers a premium to access it.
Using AI for competitive advantage
Today, the trend for many businesses is to leverage AI in smart apps and via third parties. AI technologies are widespread and easily accessible in the fields of retail, e-commerce, advertising, personalisation, logistics and customer service.
To really capture the added value of this technology however, businesses must make it a core component of their model. The good news is that you can now develop and train your own AI using a variety of open source tech and data libraries such as Google’s Tensor Flow, Kera, Spark MLlib and Microsoft’s Cognitive Toolkit. These platforms were released around 2015, which just goes to show how early we are in the journey of democratising AI. The companies that will have longevity are those that can harness their own data to optimise business added value.
The first question a company needs to ask is what are the tasks most suited to AI? It’s more than just repetitive tasks such as monthly account reconciliation for accountants or scanning at supermarket tills. That’s the niche that Robotic Process Automation (RPA) has found a footing in – but isn’t in itself a form of AI.
The second question is how can you harvest and leverage big data? Is there room to improve the quality of that data and how does that tie into your business goals? Sorting this out will help close the gap.
To get the most value from AI, companies must also consider existing processes and roles. How does it influence existing company activities, how do you manage that change? Do you have the talent needed within the team that can get the most out of AI without detracting from your current business goals? Making your business future fit with AI is about considering the talent in your team and making that adjustment over time. The gap can be especially big for businesses that are not tech first.
In 2018, we saw North American companies starting to raid universities for talent (alarm was raised about a brain-drain in Britain as Amazon and Uber whisked away Cambridge machine learning professors). This was a sign of a bigger talent problem. I recently came across a tech company that acquired a smaller US competitor solely to bring in their team of Data Scientists (a key skill set for any AI company).
One way start-ups that are building their own AI can solve this is by being open to tapping into decentralised talent. That’s central to the culture of our fintech startup Pynk, not only in our global Crowd of price predictors but in our team as well. This approach gives us access to the very best talent, wherever they may be, and opens up another conversation about the future of work in these developing fields.
Right now, companies that adopt AI into their processes and business models see a chance to gain a first-mover advantage. At its core though, this represents a desire for increased efficiency, reduced operational costs and the ability to get insights where others can’t. For example, hedge funds seeking ‘alpha’ through AI and alternative datasets. YipitData released stats last year showing that hedge funds planned to spend $1billion on alternative data in 2019 and double that in 2020. How do you think they’re extracting insights from that data? For the financial sector, which has seen significant AI adoption, value comes in decreased costs of operation, all the way to opening up new business models e.g. the use of AI and alternative data to build credit scores for lending or make accurate assessments on fraudulent behaviours.
While the benefits and potential of AI is much hyped at the moment, the companies that are preparing themselves for the future of this technology are surely the ones that will have a sustained competitive advantage, no matter their industry. While the transition will not be easy for many, bear in mind that it may well be worth the effort in the long run.
Author: Seth Ward, CEO of Pynk.io
Pynk has developed proprietary technology to combine human data sets and Ai to identify how assets move to create the world’s first people-powered investment portfolio.