
AI and Big Data: Can they guide investors through the pandemic?
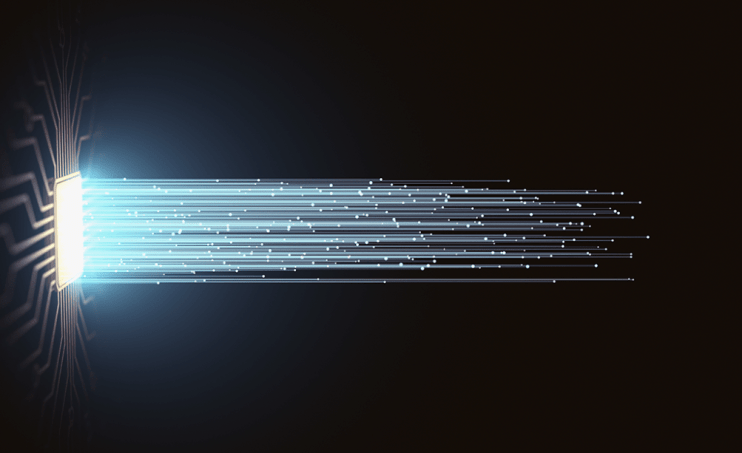
The defeat of the top human Go player by the AlphaGo artificial intelligence (AI) in 2017 revealed to the public the world of possibilities that AI scientists had been quietly exploring for years. Driverless cars, AI doctors, and robo-advisers, among other innovations, all seemed within reach.
Amid such promise and possibility, Roy Amara’s famous law was worth remembering:
“We tend to overestimate the effect of a technology in the short run and underestimate the effect in the long run.”
Indeed, the story we hear from the media three years later is more one of unfulfilled potential and disappointment at the slow pace of AI adoption than it is of revolutionary transformation.
For example, in the Economist‘s recent Technology Quarterly titled “Artificial Intelligence and Its Limits,” editors claimed that “data can be scarcer than you think and full of traps.” This limitation, among other factors, prompted them to conclude, “Some of the dreams of high summer will fade in the autumnal chill.”
This line of reasoning is as old as AI. But the global pandemic offers a rare opportunity to gauge just how well AI and big data applications in investing have performed. Since the COVID-19 crisis is of a “once-in-a-century” magnitude, researchers could not have cheated by outfitting their models with the never-before-seen pandemic period data.
So how did they manage during these tumultuous months?
Did AI programs completely fail investors? Or did they serve them well?
The easiest tests come from trading models — AI programs that predict trading signals that traders can use to decide when, where, and how to trade.
Given their short-term nature, these models tend to rely on very recent data and can rapidly adjust to changes. David Wang, CFA, who works on enhancing trading programs with AI as a managing director at State Street Bank, confirmed as much. “The low latency process we favor has performed particularly well,” he said. They also have powerful hardware to process the data very quickly.
It gets less straightforward from there. For machine learning models that need longer-term data series, new environments present a challenge. Of course, that’s hardly unique to AI programs. All quantitative models face that challenge.
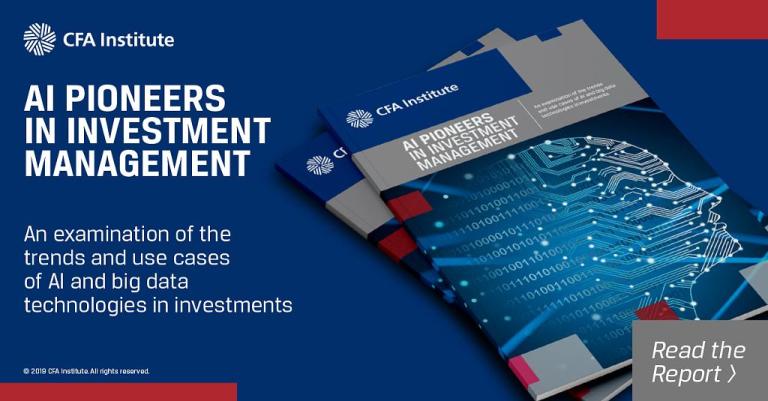
So how should investors adjust to the new data puzzle? A few options stand out, practically all of which are consistent with our philosophy that future investment teams will follow an “AI plus human intelligence (HI)” model.
AI programs are not replacements for portfolio managers and analysts but rather a source of better support. In times of crisis and uncertainty, investors will naturally rely on their experience and judgment as much as ever.
Dealing with uncertainty
The most important thing for investors to realize in times like these is that uncertainty is at the heart of this business. We have to be on constant alert for changes in the market environment. Or as Ingrid Tierens, a managing director at Goldman Sachs, put it, “All AI (and quant) models should come with a health warning of sorts.”
If we do detect changes, we should dial back our reliance on historical data. Since machine learning models are trained on data, if we don’t believe the environment from which the data was obtained is compatible with the market models, we should try simpler models.
These models would rely on fewer features, or variables that explain the output or result of the models. Reducing the number of features helps us understand what will still work in the new environment and what might not so that we are less likely to be misled by a questionable dataset.
Testing and learning
We could also check whether the ranges for the features remain broadly similar to what we previously tested. It may be a new environment but if the features remain in the same range, then our models could still hold up.
“Although recent market behavior has been volatile, the features exploited by our machine learning models were not at unprecedented levels,” said Anthony Ledford, chief scientist at Man AHL in London. “In other words, our ML models did not find themselves ‘beyond the data’ they were trained on.”
Still, Ledford added that they employ strict risk controls that reduce positions in periods of heightened volatility such as those observed recently. These are common sense best practices no matter the models or approach we apply in managing our portfolios.
Howard Marks, CFA, of Oaktree Capital, recently highlighted the critical importance of identifying regime shifts during his presentation at the CFA Institute 73rd Virtual Annual Conference. He believes Oaktree had its greatest success switching from regime to regime. This theme seems to be equally applicable to machine learning models. As Mark Ainsworth, head of data insights and analytics at Schroders, said, “If you can detect regime shifts in your model, you should be amply rewarded for it.”
Finding new opportunities to shine
What’s more encouraging for AI is that investors have gone beyond the “coping” strategies described above. They have actively pursued new applications, particularly big data applications, that help capture information in real time or at least in a more timely fashion. Tierens, for example, reported seeing increased demand for their services from the investment teams during this period. “We have been using more alternative data in the past few months than before,” she explained. “Investors understandably have more concerns in this environment, and they are all looking at alternative data because of its timeliness.”
“The pandemic really gave us an opportunity to shine as investors look to us to help explain what is happening in the marketplace,” Ainsworth confirmed. “We followed a scientist’s approach and tried to explain various development [using simpler models] rather than using classic machine learning models that fit the data, which is more typical of an engineer’s approach.”
The hype over AI brought on by AlphaGo has been fading since 2018 according to Google Trends. That’s a good sign, though, if we believe in the Gartner Hype Cycle. It simply means some pioneers have moved from hype to action. Invariably some have failed, but mainstream adoption will only take place after the “trough of disillusionment” phase shakes out the skeptics.
AI plus HI remains the overarching framework for AI adoption. As this pandemic has demonstrated, the importance of professional investors has only increased. And that shouldn’t be bad news or a disappointment to anyone.
If you liked this post, don’t forget to subscribe to the Enterprising Investor.
For more research and analysis, visit CFA Institute.
By Larry Cao, CFA is Senior Director of industry research, CFA Institute
All posts are the opinion of the author. As such, they should not be construed as investment advice, nor do the opinions expressed necessarily reflect the views of CFA Institute or the author’s employer.
Image credit: ©Getty Images / KTSDESIGN / SCIENCE PHOTO LIBRARY